The CRV program includes a number of invited speakers from all over to talk about their research programs targeting computer vision and robotics. Keynote speakers will give long talksto kick off each day. Symposium speakers will give short talks and chair each session. The confirmed speakers are listed below. Details will be udpated later.
CRV 2022 speakers (in alphabetical order) are:
Keynote Speakers
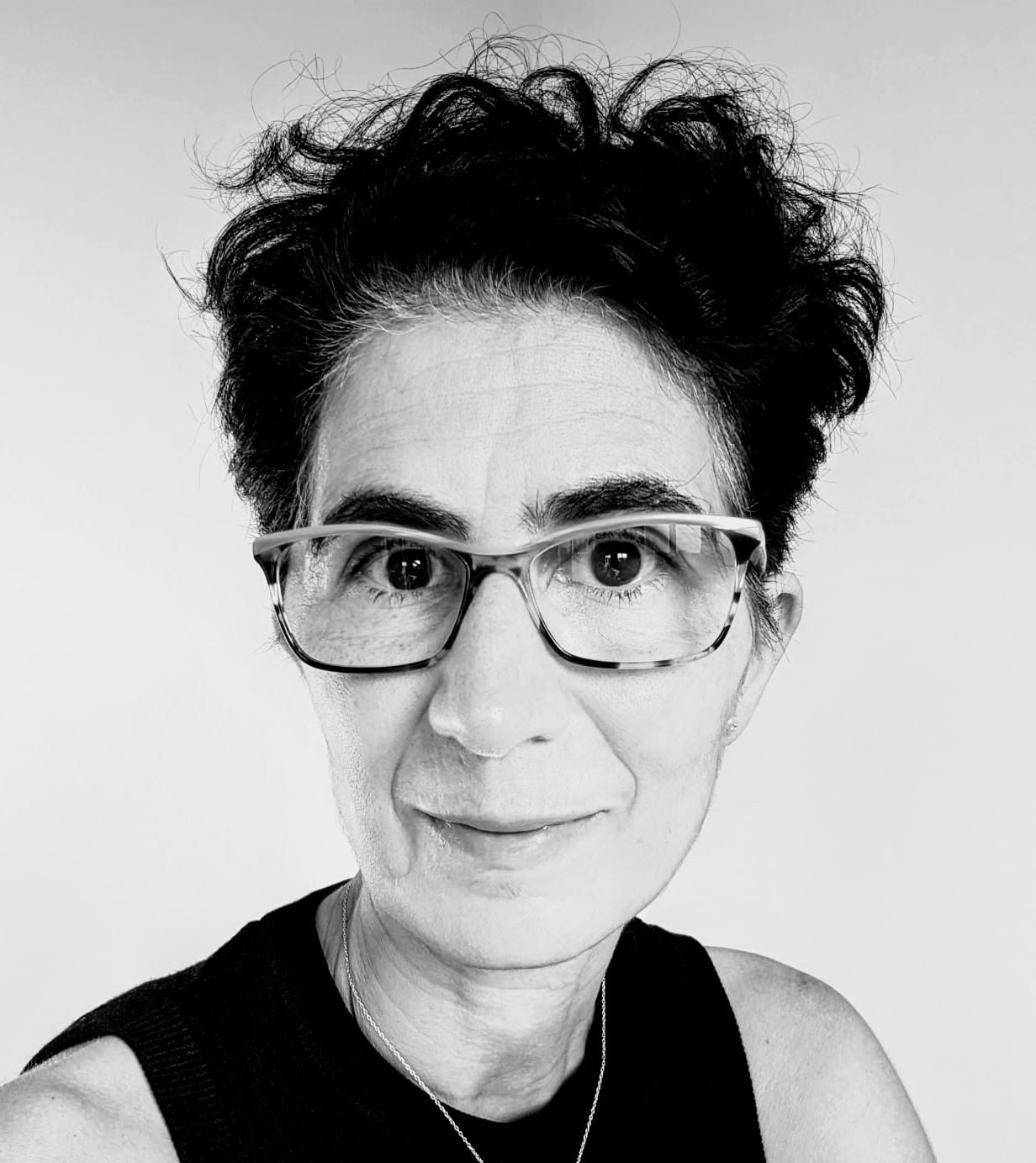
Lourdes Agapito
University College London
Talk Title: Learning to Reconstruct the 3D World from Images and Video
Abstract
As humans we take the ability to perceive the dynamic world around us in three dimensions for granted. From an early age we can grasp an object by adapting our fingers to its 3D shape; understand our mother’s feelings by interpreting her facial expressions; or effortlessly navigate through a busy street. All these tasks require some internal 3D representation of shape, deformations, and motion. Building algorithms that can emulate this level of human 3D perception, using as input single images or video sequences taken with a consumer camera, has proved to be an extremely hard task. Machine learning solutions have faced the challenge of the scarcity of 3D annotations, encouraging important advances in weak and self-supervision. In this talk I will describe progress from early optimization-based solutions that captured sequence-specific 3D models with primitive representations of deformation, towards recent and more powerful 3D-aware neural representations that can learn the variation of shapes and textures across a category and be trained from 2D image supervision only. There has been very successful recent commercial uptake of this technology and I will show exciting applications to AI-driven video synthesis.Bio
Lourdes Agapito holds the position of Professor of 3D Vision at the Department of Computer Science, University College London (UCL). Her research in computer vision has consistently focused on the inference of 3D information from single images or videos acquired from a single moving camera. She received her BSc, MSc and PhD degrees from the Universidad Complutense de Madrid (Spain). In 1997 she joined the Robotics Research Group at the University of Oxford as an EU Marie Curie Postdoctoral Fellow. In 2001 she was appointed as Lecturer at the Department of Computer Science at Queen Mary University of London. From 2008 to 2014 she held an ERC Starting Grant funded by the European Research Council to focus on theoretical and practical aspects of deformable 3D reconstruction from monocular sequences. In 2013 she joined the Department of Computer Science at University College London and was promoted to full professor in 2015. She now heads the Vision and Imaging Science Group, is a founding member of the AI centre and co-director of the Centre for Doctoral Training in Foundational AI. Lourdes serves regularly as Area Chair for the top Computer Vision conferences (CVPR, ICCV, ECCV) was Program Chair for CVPR 2016 and will serve again for ICCV 2023. She was keynote speaker at ICRA 2017 and ICLR 2021. In 2017 she co-founded Synthesia, the London based synthetic media startup responsible for the AI technology behind the Malaria no More video campaign that saw David Beckham speak 9 different languages to call on world leaders to take action to defeat Malaria.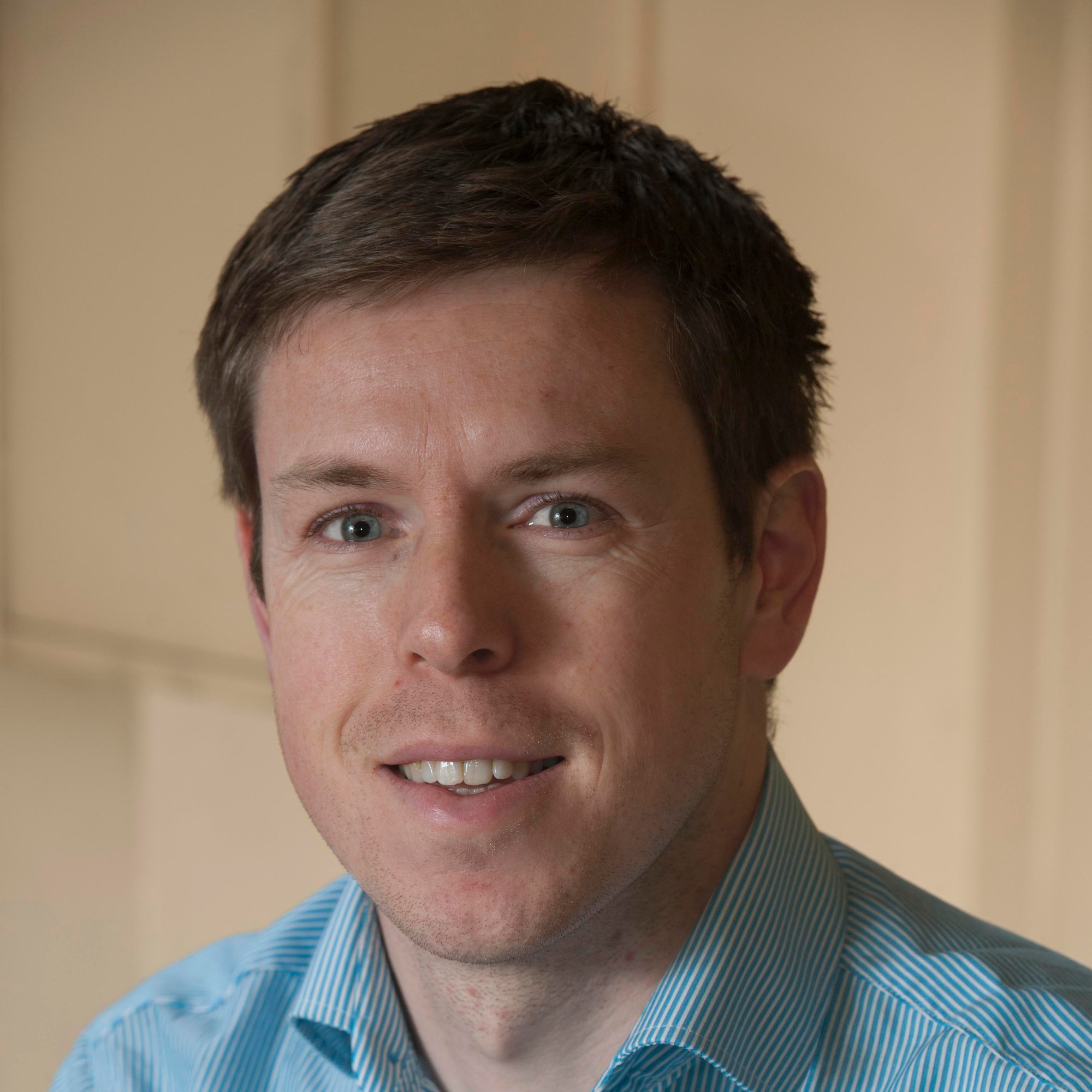
Maurice Fallon
University of Oxford
Talk Title: Multi-Sensor Robot Navigation and Subterranean Exploration
Abstract
In this talk I will overview the work of my research group, Dynamic Robot Systems Group. I will focus on multi-sensor state estimation and 3D mapping to enable robots to navigate and explore dirty, dark and dusky environments - with an emphasis on underground exploration with quadrupeds. This multitude of sensor signals need to be fused efficiently and in real-time to enable autonomy. Much of the work will be presented in the context of the DARPA SubT Challenge (Team Cerberus) and the THING EU project. I will also describe our work on trajectory optimization for dynamic motion planning and the use of learning to bootstrap replanning.Bio
Maurice Fallon is an Associate Professor and Royal Society University Research Fellow at University of Oxford. His research is focused on probabilistic methods for localization and mapping. He has also made research contributions to state estimation for legged robots and is interested in dynamic motion planning and control. His PhD was from University of Cambridge in the field of sequential Monte Carlo methods. He worked as a PostDoc in Prof. John Leonard's Marine Robotics Group in MIT from 2008 before leading the perception part of MIT's entry in the DARPA Robotics Challenge. He has worked in domains as diverse as marine robots detecting mines, humanoid robotics and mapping radiation in nuclear facilities.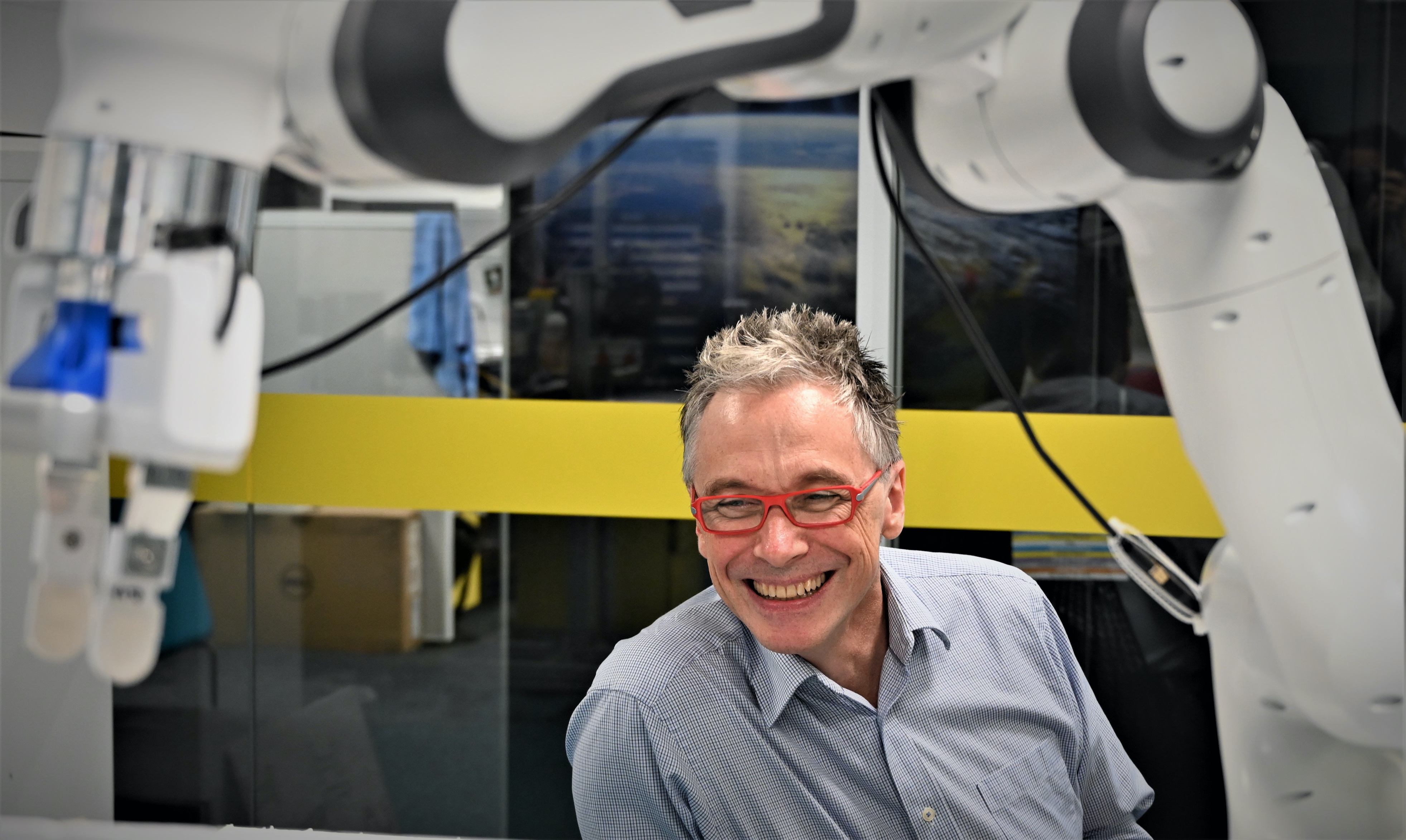
Peter Corke
Queensland University of Technology
Talk Title: Hand-eye coordination (and other things)
Abstract
Hand-eye coordination is an under-appreciated human super power. This talk will cover the robot equivalent, robot hand-camera coordination, where computer vision meets robotic manipulation. This robotic skill is needed wherever the robot’s workpiece is not precisely located, or is moving, or the robot moving. The talk will motivate the problem, review recent progress in the field, and give an update on some new software tools for robotics research.Bio
Peter Corke is a robotics researcher and educator. He is the distinguished professor of robotic vision at Queensland University of Technology, and former director of the ARC Centre of Excellence for Robotic Vision. He is a technical advisor to emesent and LYRO Robotics, and Chief Scientist of Dorabot. His research is concerned with enabling robots to see, and the application of robots to mining, agriculture and environmental monitoring. He created widely used open-source software for teaching and research, wrote the best selling textbook “Robotics, Vision, and Control”, created several MOOCs and the Robot Academy, and has won national and international recognition for teaching including 2017 Australian University Teacher of the Year. He is a fellow of the IEEE, the Australian Academy of Technology and Engineering, the Australian Academy of Science; former editor-in-chief of the IEEE Robotics & Automation magazine; founding editor of the Journal of Field Robotics; founding multimedia editor and executive editorial board member of the International Journal of Robotics Research; member of the editorial advisory board of the Springer Tracts on Advanced Robotics series; recipient of the Qantas/Rolls-Royce and Australian Engineering Excellence awards; and has held visiting positions at Oxford, University of Illinois, Carnegie-Mellon University and University of Pennsylvania. He received his undergraduate and masters degrees in electrical engineering and PhD from the University of Melbourne.Symposium Speakers
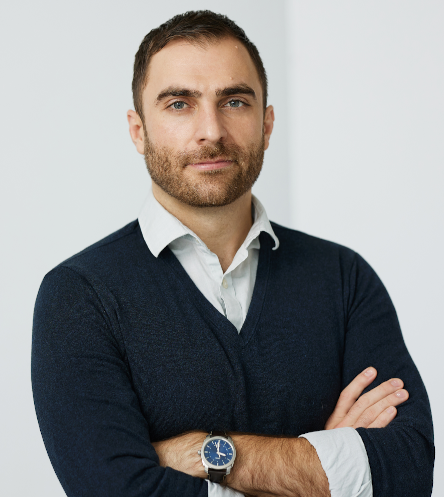
Glen Berseth
Université de Montréal
Talk Title: Developing Robots that Autonomously Learn and Plan in the Real World
Abstract
While humans plan and solve tasks with ease, simulated and robotics agents struggle to reproduce the same fidelity, robustness and skill. For example, humans can grow to perform incredible gymnastics, prove that black holes exist, and produce works of art, all starting from the same base learning system. I will present a collection of recent work that indicates we can train agents to learn these skills; however, they need to learn from a large amount of experience. To enable this learning, the agent needs to (1) be able to collect a large amount of experience, (2) train its model to best reuse this experience and (3) optimize general objectives for understanding and controlling its environment.Bio
Glen Berseth is an assistant professor at the Université de Montréal, a core academic member of Mila, CIFAR AI chair, and co-director of the Robotics and Embodied AI Lab (REAL). He was a Postdoctoral Researcher with Berkeley Artificial Intelligence Research (BAIR) working in the Robotic AI & Learning (RAIL) lab with Sergey Levine. He completed his NSERC-supported Ph.D. in Computer Science at the University of British Columbia in 2019, where he worked with Michiel van de Panne. He received his MSc from York University under the supervision of Petros Faloutsos in 2014 and worked at IBM (2012) and with Christopher Pal at ElementAI (2018). His goal is to create systems that can learn and act in the world intelligently by developing deep learning and reinforcement learning methods that solve diverse planning problems from vision.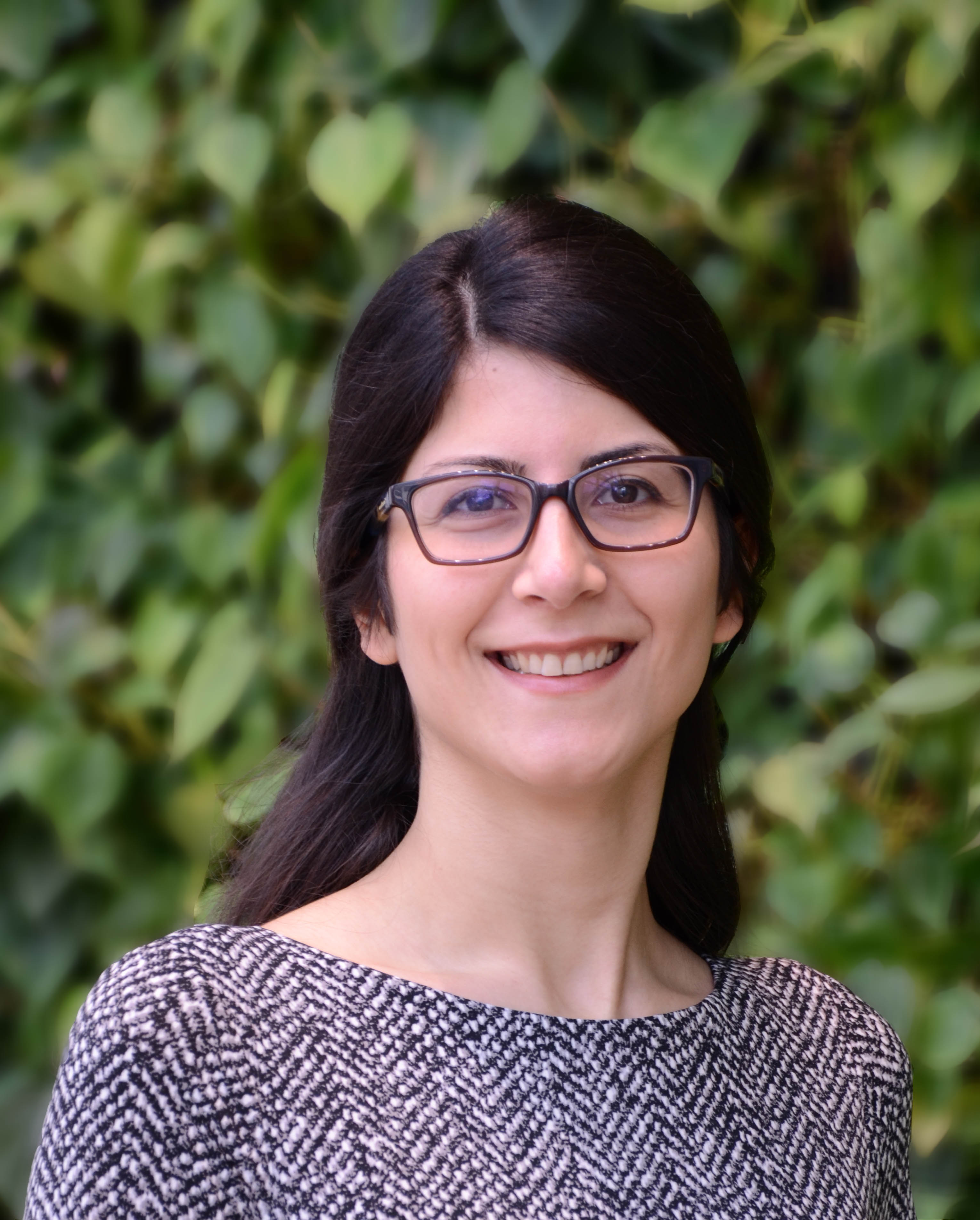
Mahdis Bisheban
University of Calgary
Talk Title: Control of UAVs in wind
Abstract
In this talk, first, I would like to present my research on the problem of estimation for rigid body dynamics. I will present a method to estimate unknown parameters of a rigid body dynamics model specifically on the Special Euclidian Group. Next, I would like to talk about a geometric adaptive controller for a quadrotor unmanned aerial vehicle with artificial neural networks. The dynamics of a quadrotor can be disturbed by the arbitrary, unstructured forces and moments caused by wind. I will show that if the control system is directly developed on the special Euclidean group and augmented with the multilayer neural networks, and the weights of the neural networks are adjusted online according to an adaptive law, we can mitigate the wind effects.Bio
Dr. Mahdis Bisheban is an Assistant Professor in the Department of Mechanical and Manufacturing Engineering since July 2021. Previously, she served as a Research Associate at the Aerospace Research Center, National Research Council Canada (NRC), and as a Postdoctoral Fellow in the Department of Mechanical and Materials Engineering, Queen’s University. Her current research focuses on two pillars: (i) Control, Estimation and Modelling of Aerial and Underwater Vehicles and (ii) Collaborative robots.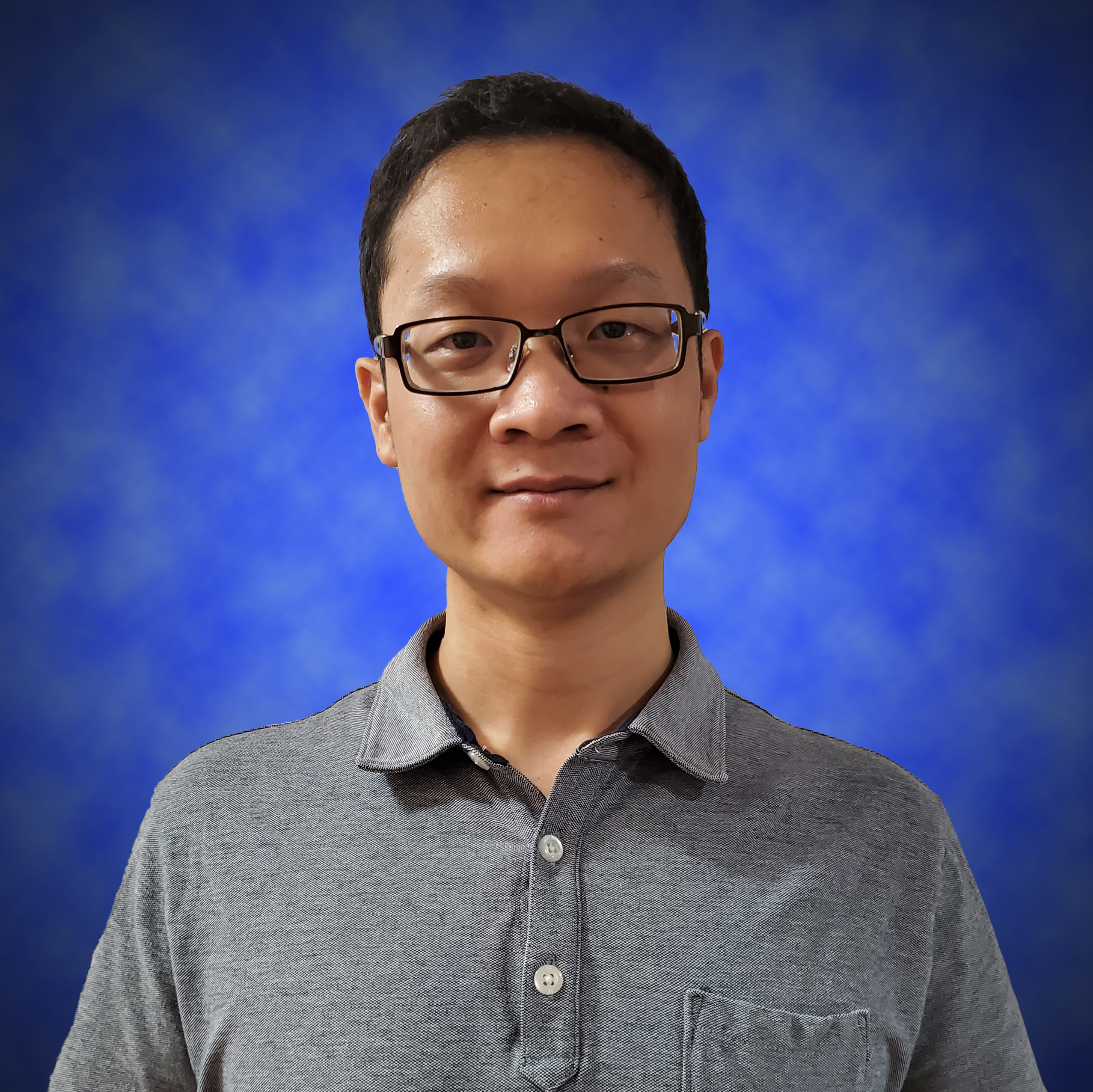
Mo Chen
Simon Fraser University
Talk Title: Control, learning, and multi-agent RL
Abstract
Autonomous mobile robots are becoming pervasive in everyday life, and hybrid approaches that merge traditional control theory and modern data-driven methods are becoming increasingly important. In this talk, we first examine how value functions and control policies obtained from control theory can improve data efficiency and generalization of robotic learning. Then, we discuss recent developments in hierarchical multi-agent reinforcement learning.Bio
Mo Chen is an Assistant Professor in the School of Computing Science at Simon Fraser University, Burnaby, BC, Canada, where he directs the Multi-Agent Robotic Systems Lab. He completed his PhD in the Electrical Engineering and Computer Sciences Department at the University of California, Berkeley with Claire Tomlin in 2017, and received his BASc in Engineering Physics from the University of British Columbia in 2011. From 2017 to 2018, Mo was a postdoctoral researcher in the Aeronautics and Astronautics Department in Stanford University with Marco Pavone. His research interests include multi-agent systems, safety-critical systems, human-robot interactions, control theory, and reinforcement learning. Mo received the 2017 Eli Jury Award for his research and the 2016 Demetri Angelakos Memorial Achievement Award and his mentorship of students.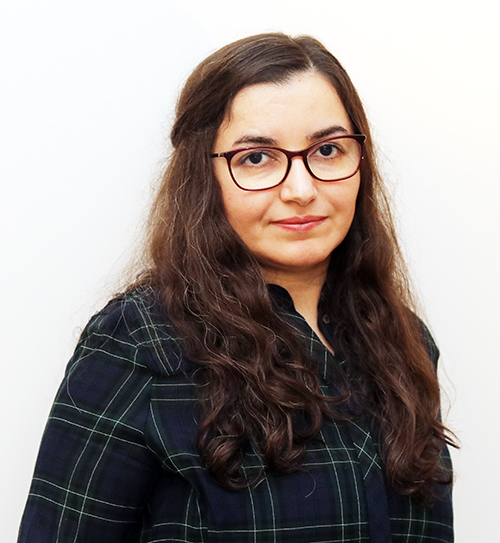
Samira Ebrahimi Kahou
ÉTS/Mila
Talk Title: Learning Dynamical Representations
Abstract
Capturing aspects of dynamics plays an important role in many decision making tasks. Some of the main challenges in learning dynamics are: uncertainty in future, modeling long-term dependencies, partial observability and learning efficient representations. In this talk, I present three works on learning dynamics, for multi-agent trajectory prediction, learning robust representations in partially observable environments, and a sequential model for designing reward in automatic evaluation of crane operators.Bio
Samira Ebrahimi Kahou is an Associate Professor at École de technologie supérieure/Vision and RL Lab. She is a Canada CIFAR AI Chair and member of Mila. Before joining ÉTS, she was a postdoctoral fellow working with Doina Precup at McGill/Mila. She received her Ph.D. from Polytechnique Montréal/Mila in 2016 under the supervision of Chris Pal. She also worked as a Researcher at Microsoft Research Montréal. Her research lab focuses on the intersection of computer vision and reinforcement learning with diverse applications.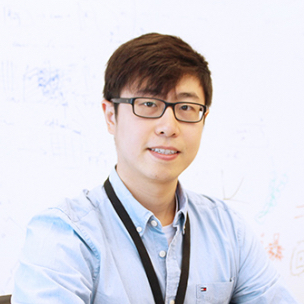
Mengye Ren
Google Brain
Talk Title: Visual Learning in the Open World
Abstract
Over the past decades, we have seen machine learning making great strides in understanding visual scenes. Yet, most of its success relies on training models on a massive amount of data offline in a closed world and evaluating them in a similar test environment. In this talk, I would like to envision an alternative paradigm that will allow machines to acquire visual knowledge through an online stream of data in an open world, which entails abilities such as learning visual representations and concepts efficiently with limited and non-iid data. These capabilities will be core to future applications of real-world agents such as robotics and assistive technologies. I will share three recent papers towards this goal, and these works form three levels in our open-world visual recognition pipeline: the concept level, the grouping level, and the representation level. First, on the concept level, I will introduce a new learning paradigm that rapidly learns new concepts in a continual stream with only a few labels. Second, on the grouping level, I will discuss how to learn both representations and concept classes online without any labeled data by grouping similar objects into clusters. Lastly, on the representation level, I will present a new algorithm that learns general visual representations from high resolution raw video. With these different levels combined, I am hopeful that future intelligent agents will be able to learn on-the-fly without manually collecting data beforehand.Bio
Mengye Ren is a visiting researcher at Google Brain and an incoming assistant professor at New York University. Previously, he obtained his PhD from the University of Toronto. From 2017 to 2021 he was also a research scientist at Uber ATG and Waabi working on self-driving cars. His research focuses on enabling machines to continually learn, adapt, and reason in naturalistic environments, and he has done a series of work on combining few-shot, semi/un-supervised, and continual learning algorithms. He has won several awards including twice the NVIDIA research pioneer award and the Alexander Graham Bell Canada Graduate Fellowship.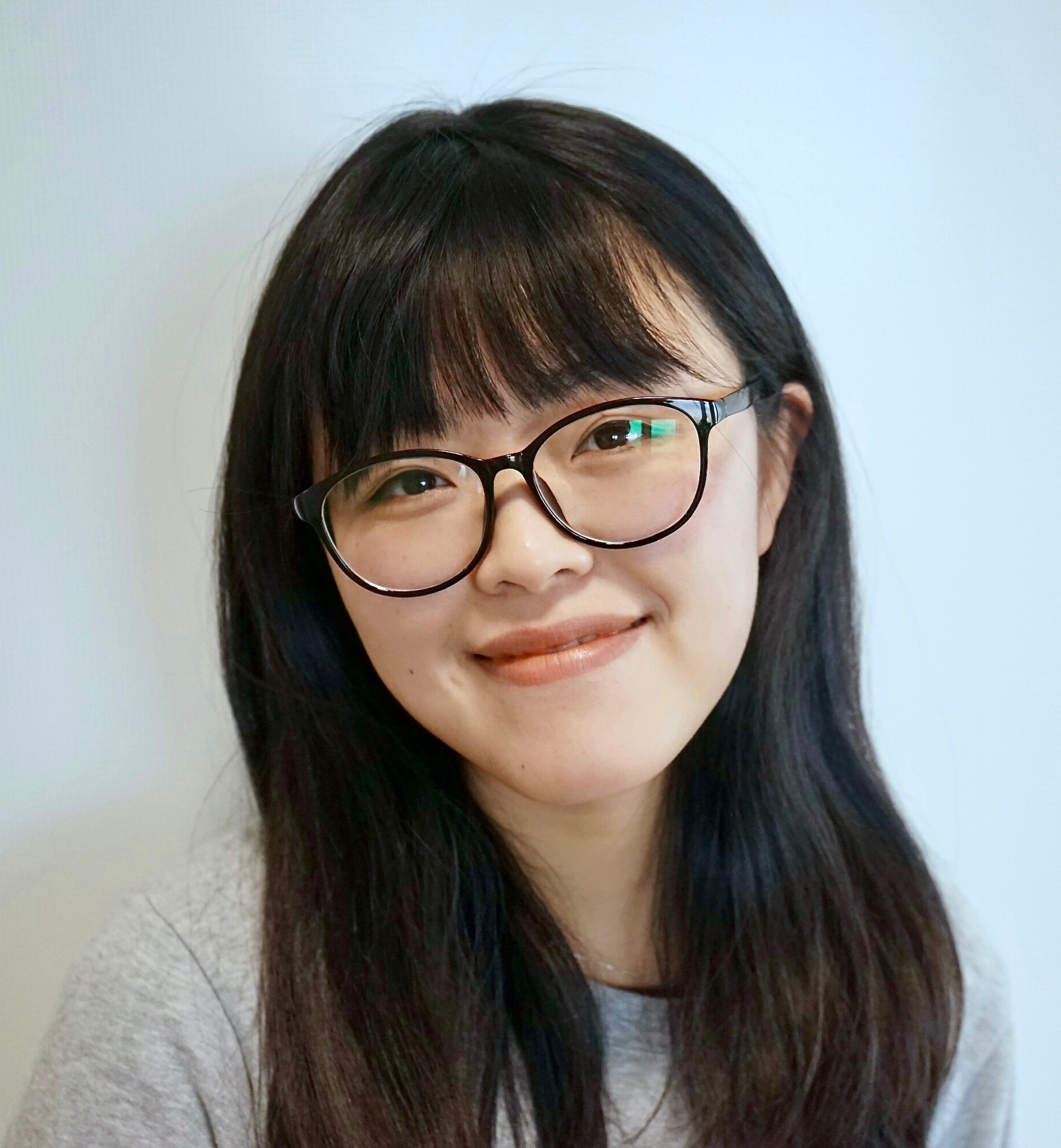